A new model reduces uncertainty in AI
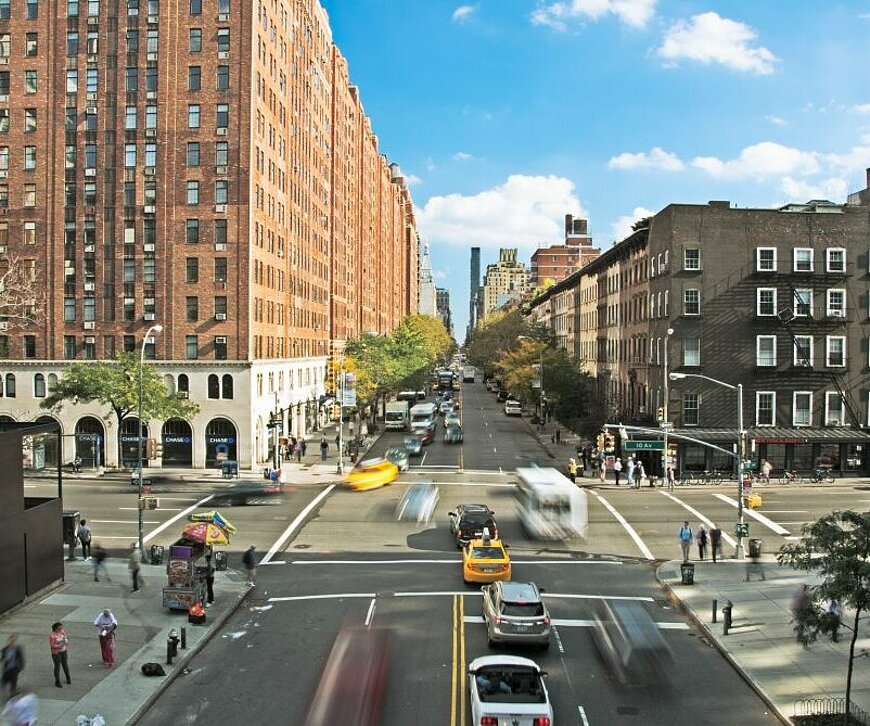
"This could take us another step further in the development of autonomous choices by computers."
How can you give uncertainties in real life a place in theoretical models? A new mathematical model from researchers at Radboud University in Nijmegen and TU Eindhoven ensures that AI can increasingly take uncertainty into account, leading to better and safer solutions. Think, for example, of a self-driving car that can make better choices at a busy intersection.
Artificial intelligence (AI) is increasingly being used to ‘calculate’ safe, affordable, and smart choices. The more complex the choices, the more computing power is required. And even then, the reality remains unruly and often difficult to predict. “There are many uncertain factors, such as the behavior of people or weather conditions. The better we succeed in predicting the real world in the model, the fewer uncertainties there are and the greater the chance that the right decisions will be made,” says Nils Jansen, principal researcher at Software Science at Radboud University in Nijmegen.
Great computing power
This is exactly what Jansen and other researchers have managed to do. They relied on uncertain Partially Observable Markov Decision Processes, or uPOMDPs, which are mathematical models that try to calculate probabilities based on real-world events. POMDPs are already used to simulate and model situations. For example, they can help to predict the spread of an epidemic, they calculate how collisions between aircraft can be avoided, and they can be used to study endangered species. Jansen: “We know that these models are good at giving a realistic representation of the world. But because of the large computing power required to use them, their use in practical applications is still limited.”
Assessing situations better
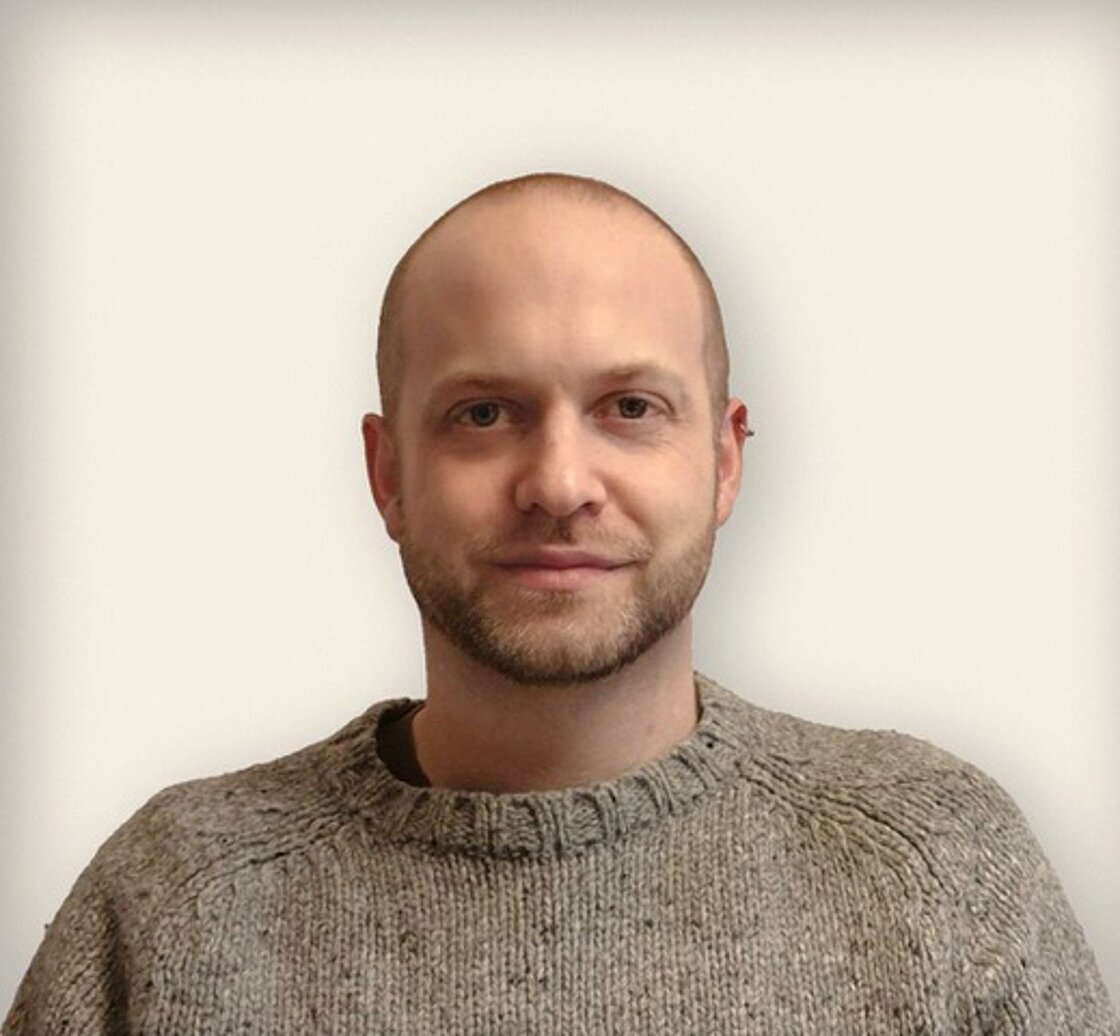
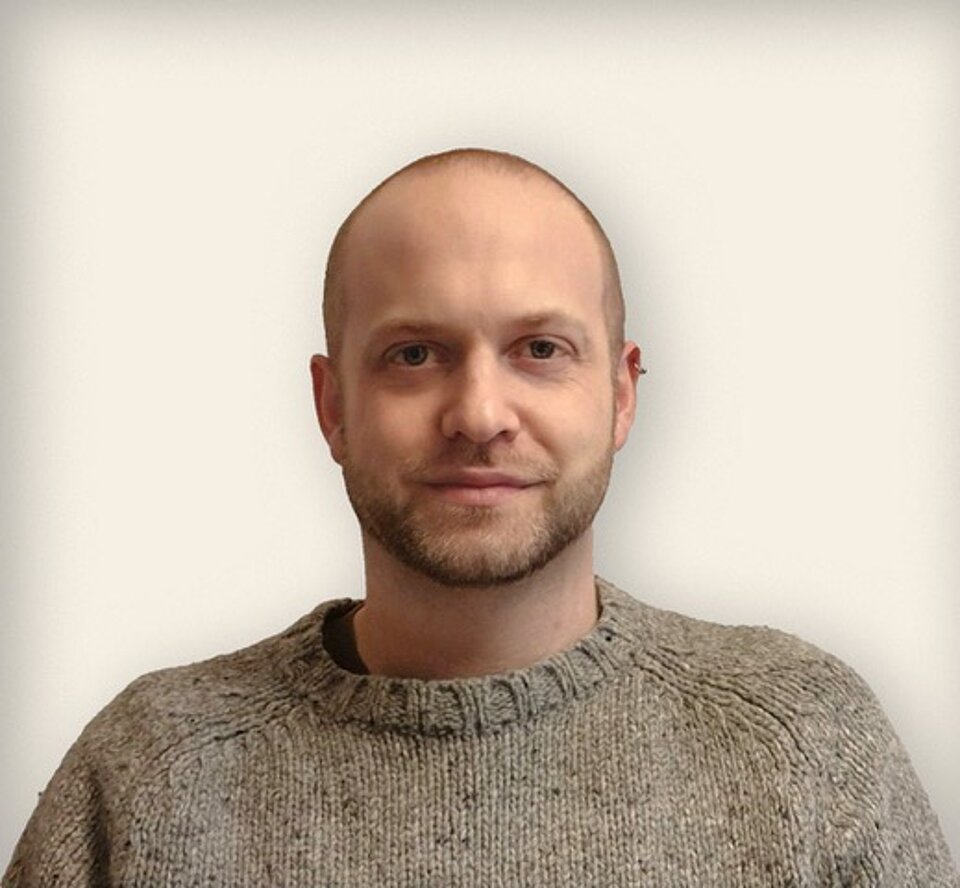
The researchers have ensured that real data from the real world can be incorporated into a theoretical model. The model continually learns from reality so that uncertainty can be reduced. The model has been used in a system to prevent collisions between aircraft, a movement planner for satellites and self-driving cars, among other things. Jansen: “Suppose the probability that a self-driving car correctly assesses the traffic situation is about 90 percent. Then it is still uncertain what risks you run in the remaining 10 percent. And even in that 90 percent there is still a margin of uncertainty. All in all, that’s an unclear and vague approach to risk. In such a situation, you don’t want to expose someone to the rather uncertain choices that a self-driving car makes. With this new approach, a system would be able to provide much more detailed explanations of what happens in practice and take that into account when making new calculations. For users, this means having more specific information about what could go wrong. There are no guarantees, but the decision becomes more robust by, for example, letting the car stand still for a little longer and assessing the situation before deciding to cross the busy intersection. With more adequate models you are better able to avoid risk and uncertainty.”
Open source model
Working with uPOMDPs has previously been considered by other research groups. Jansen: “It was considered a difficult problem, but thanks to an interdisciplinary approach with researchers from different countries, we were able to force a breakthrough. For the first time, we have now been able to turn previous theoretical thought experiments into a practical and realistic approach.” The research team in Nijmegen and Eindhoven collaborated with researchers at the University of Texas (Austin) and the University of California (Berkeley) on this project.
The new model will be presented at the AAAI Conference on Artificial Intelligence in early February and will then be free to use by companies working with AI predictions. “It’s applicable in all sorts of areas. For example, you can predict when you need to have maintenance done on your machines or car,” says Jansen. “At least you want to be on time and not late because your car doesn’t work anymore. With this model, you can reduce the uncertainty.”
Opportunities in practice
Arno van der Steen, project leader Automotive Research and expert at the Advanced Mobility minor at the Hogeschool Arnhem Nijmegen (HAN), sees possibilities for this new model. “The beauty of this kind of model is that we can calculate more different situations with less computing power and reduce uncertainty. If you now want computer models to make all decisions at the right time and in the right form, then this is impossible in terms of computing capacity. Developments in computing capacity and storage are going fast, but not yet so fast that we can let a self-driving car take all the decisions. Having a car drive between the lines on a highway by itself is quite feasible nowadays. And by increasing computing capacity and eliminating uncertainties, you can slowly let computers take over more and more. But it will take some time before we can let the car decide for itself at a busy intersection where people are making unpredictable choices. I don’t know the new model developed by the researchers yet, but I can imagine that this could take us another step further in the developments of autonomous choices by computers.”